AI Hallucination Prevention Specialist
I help engineering leaders eliminate AI hallucinations and prevent $50k+ in losses. Fast.
Your $50,000 AI is worthless if it hallucinates publicly.
In 7 days, get your customized roadmap for permanently stopping AI hallucinations and deploy with confidence.
Stop AI Hallucinations. One hour, one call, clear roadmap. $400.
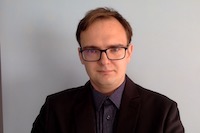
The #1 Reason Your AI Implementation Fails
Most AI systems fail in production because of three critical evaluation gaps:
Data Drift
Your team is testing with artificial data that doesn't represent real-world conditions
Pattern Recognition Gaps
Your engineers can't identify what's causing incorrect outputs
Missing Domain Expertise
Subject matter experts weren't involved in the development process
The Fastest Way to Fix AI Hallucinations
In just one hour, we’ll pinpoint the cause of your hallucination issues—and within 7 days, you'll receive a custom, production-ready roadmap to eliminate them.
🎯 What You Get
- ✔️ One-hour 1:1 video consultation
- ✔️ Review of your current AI system’s hallucination triggers
- ✔️ Tailored hallucination prevention roadmap (delivered in 7 days)
- ✔️ Practical next steps your team can implement immediately
- ✔️ Direct access to my 14+ years of implementation expertise
- ✔️ Optional: Implementation support available after the meeting
💰 Investment
One-time fee: $400 USD
Walk away with a clear roadmap—or let me help you implement it. You choose.
One call. One week. One roadmap to prevent hallucinations in production.
Proof: Results in Real Production Environments
Here's what happened when I applied my methodology:
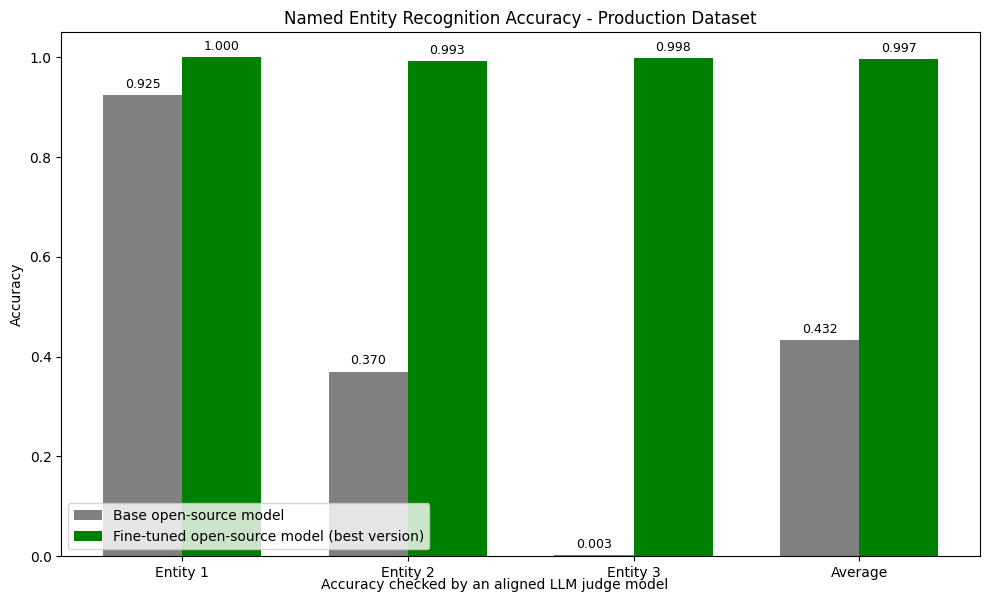
Before: 57% error rate
After: 0.3% — achieved in just 4 days.
Why Work With Me?
AI hallucinations are not just technical bugs they’re business risks. I bring a rare combination of hands-on AI experience, engineering leadership insight, and instructional clarity.
- ✅ 14 years writing production-ready code that doesn’t fail under pressure
- ✅ MLOps & data engineering experience in systems where accuracy wasn’t optional
- ✅ Trained 1,006 engineers to build AI systems that actually work in production
- ✅ Speaker, author, and creator of practical tools—used by real-world teams
You're not hiring a junior consultant or theory-heavy academic. You’re working directly with someone who’s solved this in production under pressure.
What Engineers Say:
"I'm extremely impressed with Bartosz's expertise and experience. We covered all assignments, addressing various details, scenarios, and potential errors. Every question we asked was answered thoroughly."
"The training was exceptional because it successfully integrated theory with practical application. The exercises allowed us to immediately implement what we had learned, and the real project examples were incredibly useful."
"Everything was conducted excellently. A considerable amount of knowledge but presented gradually so that it was clear what led to what."
Don’t Let AI Hallucinations Ruin Your Launch.
One hour to clarity. Seven days to eliminate risk. No fluff—just real results.
Book Your ConsultationOne call. One week. One roadmap to prevent hallucinations in production.
Recent Articles on AI Implementation Reliability:
How I Transformed a Failing AI System into 99.7% Accuracy in 4 Days (And Eliminated a €20M Regulatory Risk)
Learn how to implement production-ready AI systems using a systematic approach focusing on error analysis rather than complex models. This case study shows how to transform unreliable AI prototypes into trusted production systems that eliminate regulatory risks and deliver real business value.
How to Make AI Evaluation Affordable: Research-Backed Methods to Cut LLM Evaluation Costs
Why are your AI evaluation costs spiraling out of control, and what are the proven methods to reduce them without sacrificing quality?
The Hidden Reason Your RAG System Is Failing - The Problems Caused by Approximate Nearest Neighbor Search in Vector Databases
Discover why your RAG system might be failing due to Approximate Nearest Neighbor search limitations in vector databases. Learn how compute budgets affect search accuracy, why metadata filters complicate retrieval, and implement practical solutions to dramatically improve your RAG performance.
Why is it so hard to correctly estimate AI projects?
Why can't you estimate an AI project correctly and can you do anything about it?
Building Reliable AI: A Testing-First Approach
Learn how to properly test AI systems using familiar software testing concepts. Discover key metrics, alignment checks, and robustness testing strategies for reliable AI deployment.
From API Wrappers to Reliable AI: Essential MLOps Practices for LLM Applications
API wrapper or production-ready AI? Learn how proper LLMOps separates prototypes from reliable applications
Troubleshooting AI Agents: Advanced Data-Driven Techniques of Improving AI Agent Performance
Expert strategies for improving AI agent performance through better data retrieval, query generation, automated decision-making process, and response generation. The article covers data collection, metrics, and techniques to improve the agent's performance.
Comprehensive Guide to AI Workflow Design Patterns with PydanticAI code examples
Learn how to implement AI workflows and autonomous agents with PydanticAI. This guide shows an example implementation of patterns described in the Anthropic article 'Building effective agents' such as prompt chaining, routing, parallelization, and orchestrator-workers.
How Much Data Do You Need to Improve RAG Performance?
A data-driven approach for improving RAG performance. Learn how to gather data and how much data you need for RAG, fine-tuning LLM, and training a specialized LLM from scratch.
The Ultimate 2025 Guide to Prompt Engineering
Discover the difference between proven prompt engineering techniques and tricks