Running a business in the franchise model poses a unique set of challenges. You can’t be everywhere, and you have a limited impact on the quality of service, but at the same time, every interaction with a franchise impacts the customer’s opinion about your entire brand.
Table of Contents
- The challenge: How do we extract insight from user reviews?
- The AI-powered review analysis and reporting solution
It gets even worse when you run bakeries. Generally, customers won’t give you a second chance. If they don’t like your product, they will go somewhere else. A one-time mistake is already too much. After all, if a tasteless cake ruins someone’s birthday, they won’t buy anything from the bakery that made the cake ever again.
The challenge: How do we extract insight from user reviews?
As in every business, bakery customers write online reviews. As in every industry, most satisfied customers stay silent, and only people with something to complain about write reviews. That makes every happy customer who wants to spend time writing a review even more valuable and every dissatisfied customer a tragedy.
Reading all of the reviews yourself is feasible only when you own a single shop. The people who run the franchises can read their reviews, and we hope they do, but for the entire brand, we need something better. We need something that can not only deliver actionable insight but also distinguish between issues related to a single shop or the entire brand.
For the sake of this article, I prepared a test dataset of reviews of non-existing bakeries. None of the data shown here belongs to an actual business.
The AI-powered review analysis and reporting solution
The implemented solution used AI to assign customer reviews to categories we cared about, extract relevant quotes from the text, determine whether the review is positive or negative based on the text (because sometimes people don’t understand the star-rating system and give you 1/5 with a terrific review), and distinguish between comments about a particular location, product, or the entire brand.
Building blocks of the solution
First, we had to decide what we cared about. Every review can be assigned to several categories, and we needed to define those because letting AI do it would make creating a summary report impossible. If we didn’t limit the set of categories to a predefined list, we would end up with things that mean the same but are worded differently.
For the positive categories, we choose around twenty categories. The list contained things such as “Friendly Staff,” “Great Ambience,” “Wheelchair Accessibility,” and, of course, “Tasty Pastries” or “Excellent Presentation.” For the negative reviews, we had a slightly longer list with issues such as “Slow Service,” “Poor Hygiene,” “Cash Only,” “Inconsistent Quality,” etc. Because some locations offered delivery, we also created category lists for reviews related to deliveries.

Result delivered as a single-page report
While displaying review categories with ratings is already a significant improvement over reading all the reviews to gauge the condition of a franchise, we don’t need to stop there.
We can deliver a summary of reviews as a report page for every location, showing its highlights and common issues. The page tells you exactly what needs to improve, according to the reviewers, and in which customer service areas a particular bakery may be a role model for other franchisees.
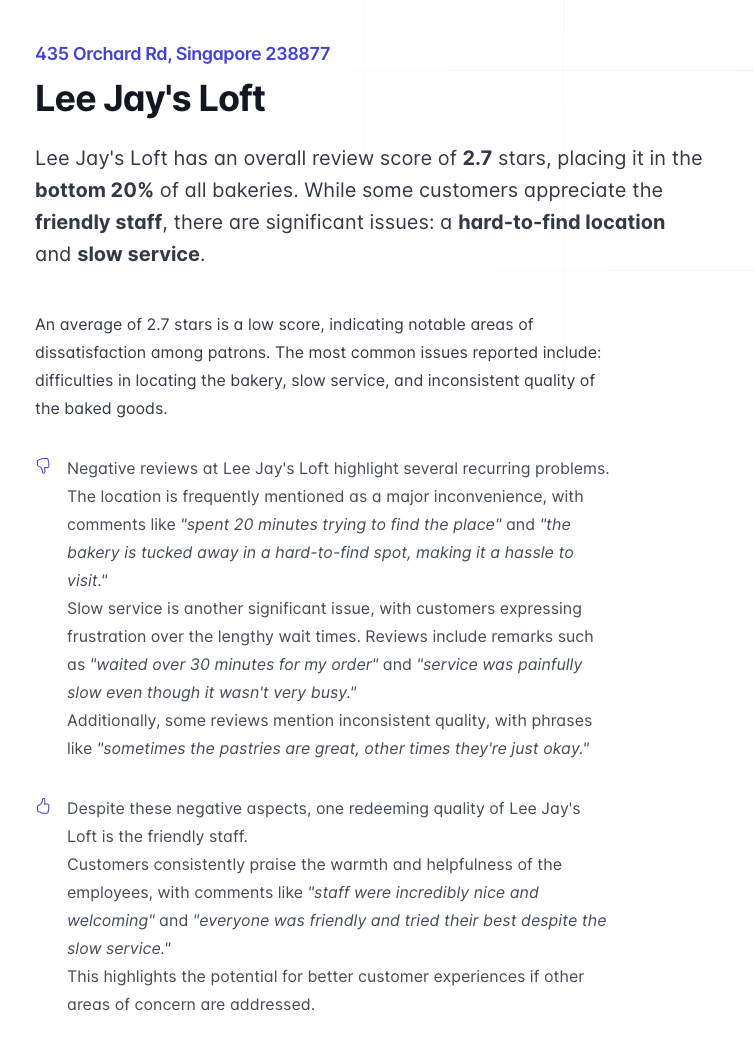
Is your AI hallucinating in production? Take my 10-minute AI Readiness Assessment to identify critical vulnerabilities or schedule a consultation.